For some time, just about every industry was raving about the potential of Big Data – the process of analyzing enormous data sets to discover patterns and trends that can then be used to guide business decisions. In the world of HR, the discourse on Big Data became so prevalent that the term started to be used as a catch-all description for any type of predictive analytics in the hiring process. But long before the concept of “Big Data” took off, companies who favored data-driven, evidence-based hiring methodologies were using pre-employment tests to gather information on prospective employees. And while pre-employment testing may be an older, more established way of gathering data on job candidates, it differs in several critical ways (both ethical and practical) from Big Data.
But before exploring the differences between tests and Big Data, let’s define our terms. When we discuss pre-employment tests, we’re referring to standardized assessments that measure various job-related abilities, skills, or traits. The most common types of tests are aptitude, skills, and personality tests. “Big Data,” as it applies to hiring, draws upon large quantities of data from a multitude of sources to form conclusions about a job candidate. These sources might include social media profiles, meta-data associated with an applicant’s employment application, and other elements of an applicant’s (increasingly long) digital footprint.
On a basic level, tests and other forms of predictive analytics have something in common: both gather information on applicants and use that information to predict business outcomes (employee performance). The similarities between tests and predictive analytics have resulted in the two being improperly conflated in the popular press. For example, several years ago there was a slew of articles in places like the Economist, The Wall Street Journal, and The Atlantic about the rise of data-driven hiring. These articles had predictable undertones of angst about algorithms taking over hiring decisions—the WSJ article was titled “Meet the New Boss: Big Data.”
One “Big Data” company named Evolv, a predictive analytics startup since acquired by Cornerstone OnDemand, was cited by all three articles - a tribute to its PR team if nothing else. The articles outlined the innovative ways Evolv helped their customer Xerox refine its hiring process. Using evidence-based techniques that included personality and cognitive tests but also information gleaned from the online application and from other digital sources, Evolv had constructed algorithms that improved Xerox’s hiring results.
Like many Big Data algorithms used for employee selection, Evolv’s algorithm scraped social media profiles along with many other elements of a candidate’s digital footprint. Through working with Xerox, Evolv apparently found that the number of social media profiles and the type of browser a candidate used were both predictive of work success.
Unfortunately, there are at least two problems with using this kind of data to make projections: one ethical and one practical/scientific in nature. On an ethical level, there is absolutely no transparency to the exchange of data from candidate to employer. The candidate is likely not aware that their social media activity is being used in a hiring algorithm, and with the possible exception of LinkedIn, their social media accounts were not created for that purpose. Unlike pre-employment tests, which involve a transparent exchange of data in which the candidate is fully aware they are being evaluated, Big Data mines many elements of a person’s digital footprint without their consent and without revealing which data may be used, or in what way. We should be careful to distinguish, then, between the different ways of gathering data on prospective employees. The first type (testing applicants) is overt, and the other (scraping data from a variety of digital sources) is covert, as a candidate has no clue their data is being mined. The latter is ethically problematic, at the very least.
The second problem with the methodologies used by Big Data is more practical in nature, and has to do with the old problem of correlation not equaling causation. Take the browser example described above. Evolv’s data scientists found that candidates who used browsers other than Internet Explorer were more likely to be high performers, and speculated that this might reflect a personality trait related to decision-making, because they made a conscious decision to download a browser other than the one that came preloaded on their computers.
Several years ago, we published an analysis of aptitude test scores by browser and concluded that there were indeed differences in aptitude scores by browser: non-Internet Explorer users scored significantly better on aptitude tests. So it was not surprising to us that Chrome and Safari users performed better on the job at Xerox, because internet browser choice is correlated with cognitive aptitude, and cognitive aptitude is one of the strongest drivers of work performance.
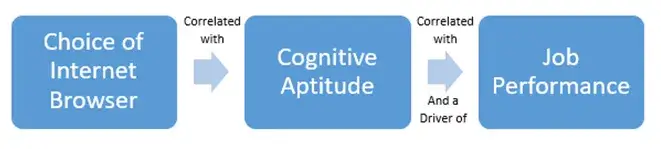
If the underlying correlation that Evolv noticed was that, in fact, users of certain browsers tend on average to be better problem-solvers and critical thinkers, then why not try to measure such aptitudes directly through assessments? If the choice of internet browser is correlated with aptitude, and aptitude is correlated strongly and directly to job performance, why not just test for cognitive aptitude instead? Doing so would not only provide a stronger predictive signal but also cut out some of the ethically gray uses of candidate data. The predictive accuracy would undoubtedly be improved, and Internet Explorer users would not be unfairly hindered in their job search.
What’s more, lessons in Big Data have shown that many indirect correlations, like the browser example, can be surprisingly fickle. One study may find a correlation and another may not. In contrast, the long and successful history of psychometrics provides confidence that skills and aptitude tests will continue to show robust associations with job performance. Who can possibly guess what kind of browsers people will be using to apply for jobs 10 years from now, and what will it signal about their prospective value to a company?
The distinction between covert and overt data-gathering is a big one, kind of like the difference between telling someone you are listening to what they are saying versus just tapping their phone without their knowledge. Well-validated pre-employment tests measure established constructs that have been shown to predict performance in organizational settings. Big Data is certainly very efficient, but risks chasing correlations at any cost, often not stopping to consider the causes, or the ethical implications of the algorithms they are constructing. Even if testing is a part of what many Big Data firms do, we should be careful not to conflate these two very different means of gathering data on prospective employees.